"It’s easy to lie with statistics, it’s hard to tell the truth without statistics.”
– Andrejs Dunkels
Reflecting on when I first started in digital marketing, this quote perfectly captures my journey. I was overwhelmed by a sea of metrics—conversion rates, ROAS, CPL—it was hard to know where to focus. Over time, I realized metrics alone are misleading. Leaning into statistics, specifically Z-scores, allowed me to uncover the truth behind the numbers, pinpointing exactly when and where to optimize my campaigns and making data truly work for me.
Using Z-scores as part of my process has helped me scale revenue volume by over 51.4% for 7 figure business and generate upwards of $21,000 in revenue from organic content.
What are Z-scores and how to use them?
Statistics was never my favourite subject—I avoided it throughout high school and university. It wasn’t until I saw its value in marketing that I decided to dive in. Don’t worry, I’ll keep this simple so you can easily replicate the process I use. So, what are Z-scores? Simply put, they measure how far a data point is from the average in a dataset.
What if a data point is far from the average? That data point is considered an anomaly.
What if a data point is close to the average? That data point is considered normal.
But how do you know when a data point is close or far enough to be normal or an anomaly? This is where the bell curve comes in handy. A bell curve, shaped like a hill, shows how values are distributed: most are clustered in the middle (the average), while fewer appear at the edges (the anomalies). For example, if we analyzed the volume of conversions across campaign channels, most channels would likely fall in the middle, generating a typical amount of conversions assuming you have tracking and everything else set up correctly. However, channels with extremely high or low performance would stand out as less common, appearing on the edges of the curve.
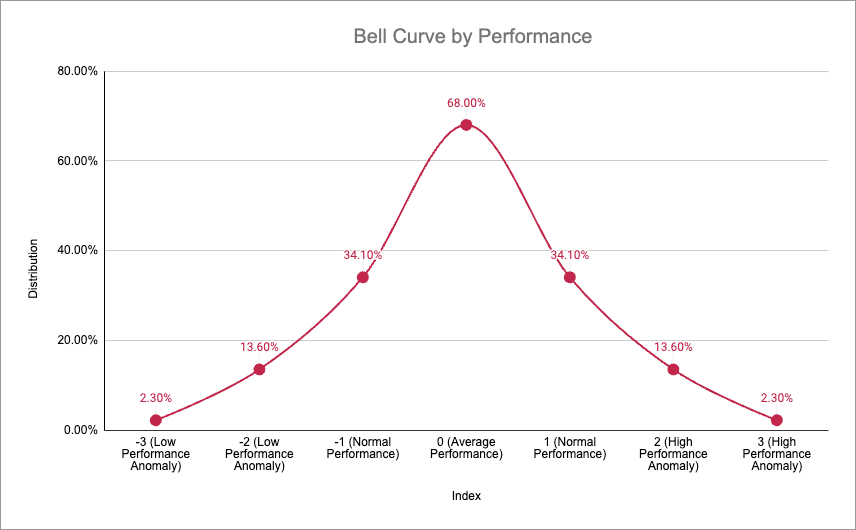
It doesn't matter whether you are comparing Facebook Ads to Performance Max, or any combination of channels. You can mix in any number ads or campaigns from any platform, the framework is designed to standardize the results for the simplest comparison. I recommend using Google Sheets or Excel when you give this your first try.
Example of Z-scores for a marketing campaign
Below is an example of a campaign I created to outline the steps for conducting a Z-score analysis and provide optimizations insights. The process consists of three main steps: the Raw Data Table, the Average and Standard Deviation for each metric, and the Z-score Data Table. Each step builds on the previous one, and by the end of this section, you’ll have the needed steps to make construct your own Z-score analysis using your campaign data and make informed decisions.
Raw Data Table
Channel | Leads | Budget Spent | Cost Per Lead |
Facebook Audience | 8 | $2,000.00 | $250.00 |
Facebook Retargeting | 15 | $1,500.00 | $100.00 |
Google Search | 20 | $3,000.00 | $150.00 |
Performance Max | 17 | $2,500.00 | $147.05 |
For this example, I focused on three metrics, but you can include as many as you need. Additional metrics worth considering are clicks, impressions, CTR, revenue, ROAS, and more. To begin building the framework, you’ll need to calculate the standard deviation and average for each metric. These values serve as the foundation for our Z-scores:
Standard Deviation: measures how much the data varies from the average.
Average: the sum of all values divided by the total number of results in the dataset.
Average and Standard Deviation for each metric
Z-score components | Leads | Budget Spent | CPL |
Standard Deviation | 5 | $645.50 | $63.13 |
Average | 15 | $2,250.00 | $161.76 |
If your data is in an Excel sheet, I recommend using built-in formulas to calculate the standard deviation and average for each metric. Calculating standard deviation manually can be quite complex, so leveraging these formulas will save time and reduce errors. Feel free to use the values from the Raw Data table to practice creating your own version of the Average and Standard Deviation for each metric. Below are the formulas to use:
Standard Deviation: in Google Sheets or Excel, use "=STDEV(B2:B5)"
Average: in Google Sheets or Excel, use "=Average(B2:B5)"
Z-score Data Table
Channel | Leads | Budget Spent | CPL | Totals |
Facebook Audience | -1.37 | -0.39 | 1.40 | -0.36 |
Facebook Retargeting | 0.00 | -1.16 | -0.98 | -2.14 |
Google Search | 0.98 | 1.16 | -0.19 | 1.96 |
Performance Max | 0.39 | 0.39 | -0.23 | 0.55 |
Totals | 0.00 | 0.00 | 0.00 | 0.00 |
We’ve reached the final step of the process: calculating the Z-score for each channel and metric. Below, I’ve outlined a step-by-step guide to show how it works and how each value can inform your decision-making process. Follow the steps below:
To calculate the Z-score, use the formula: (X - Metric Average) / Metric Standard Deviation
X: The raw data value for a specific channel and metric (e.g., Facebook Audience and Leads).
Metric Average: The average we previously calculated for the metric (e.g., Leads).
Metric Standard Deviation: The standard deviation we calculated for the metric (e.g., Leads).
Example: For Leads and Facebook Audience: (8 - 15) / 5 = -1.37
As you calculate Z-scores, the totals at the bottom rows and right columns will provide valuable insights:
Totals Row: The sum always equals zero because the positive and negative deviations from the mean balance out.
Totals Column: While not traditionally used in statistics, calculating column totals offers insights into what influences campaign performance, making it a useful addition for marketing analysis.
Here are three actionable insights from the example table:
Facebook Retargeting:
Total Z-Score: -2.14, primarily due to low budget allocation and CPL (-1.16-0.98)
Recommendation: Increase the budget by one standard deviation ($645.50) to improve performance.
Facebook Audience:
Total Z-Score: Indicates poor performance due to fewer leads and a high CPL.
Recommendation: Reduce the budget by 1 standard deviation ($645.50).
Google Search:
Total Z-Score: Indicates strong performance with CPL below the overall average.
Recommendation: Maintain the current budget or increase it by 0.19 standard deviations ($645.50 x 0.19 = $122.64).
Take some time to analyze the Z-score table yourself and explore additional insights. This framework allows for tailored recommendations to optimize campaign performance.
How and Why should you use Z-scores?
If you got this point, I hope that my explanation of what Z-scores are and how to use them proved useful for you and your campaign analysis. It's a neat framework that is standardized, easy to communicate with, and from my first hand experience it has helped scale results for clients. There is so much more to explore with this concept that I didn't expand on here but I will in future blogs and tutorials.
As to why you should be using Z-scores, I recommend using the step by step process I provided and as you get more familiar with it add other metrics or reorganize the data. After all, it's hard to tell the truth without statistics so I strongly believe that this framework is a tool to help anyone gain additional insight that would otherwise be difficult to reach by analyzing raw metrics alone. More importantly it's a tool that has proved key in my ability and my teams ability to scale results.
Comments